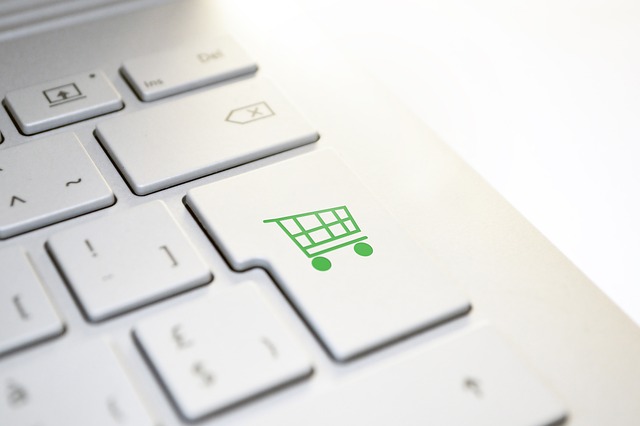
E-commerce, Ecology, and Continuous Improvement
Explore the economic impact of poor retail inventory management & a French law on unsold goods, with e-commerce’s ecological shift.
In the intricate world of supply chains, where efficiency is paramount, can AI and advanced forecasting be the answer to the challenges faced in material replenishment?
This essential process, while pivotal, is no stranger to difficulties. Factors such as varying lead times and demand fluctuations persistently challenge the status quo. These complexities often lead to production delays, increased costs, and customer dissatisfaction.
Forward-thinking organizations are now using Artificial Intelligence (AI) and advanced forecasting techniques to address these complexities.
In this article, we explore the pivotal role of forecasting, AI, and data precision in revolutionizing material replenishment.
At the core of effective material replenishment lies forecasting. Advanced AI forecasting is the cornerstone of timely material availability. In an era marked by supply chain uncertainties, traditional forecasting methods often fall short. Thus, there is a pressing need for advanced forecasting techniques.
As McKinsey reports, ‘Applying AI-driven forecasting to supply chain management, for example, can reduce errors by between 20 and 50 percent—and translate into a reduction in lost sales and product unavailability of up to 65 percent.’ This highlights the substantial impact that AI can have in improving supply chain management.
AI-driven forecasting tools leverage historical data and employ machine learning algorithms to predict future demand patterns. By identifying hidden patterns and trends, AI enhances the precision of forecasting. This enables organizations to make data-driven decisions and avoid disruptions caused by inaccurate predictions.
One of the remarkable capabilities of AI is its ability to detect subtle and complex patterns within vast datasets. Unlike conventional methods, AI-driven systems excel at uncovering correlations that might escape human analysis. This capability allows for more accurate demand forecasts and better material replenishment planning.
More and more start-ups are now entering the arena, offering AI and Machine Learning solutions that go beyond conventional Demand Replenishment Planning (DRP) models and Advanced Planning and Scheduling (APS) software. These innovative solutions have a unique focus on detecting anomalies by learning from past situations that are potential risks of stock-outs.
AI’s remarkable capability to identify subtle and complex patterns within vast datasets sets it apart from traditional methods.
Looking ahead, the future of material replenishment holds great promise, especially with the integration of Intelligent Material Management Solutions (IMMS). AI-powered forecasting, in conjunction with IMMS, empowers businesses to confidently navigate the complexities of modern supply chains.
This convergence of advanced forecasting, AI, and the precision offered by IMMS is reshaping material replenishment, ensuring its efficiency and effectiveness in an ever-evolving landscape.
Flowlity, an IMMS AI-driven solution is at the forefront of implementing the concepts discussed in this article. Flowlity’s innovative approach harnesses the power of AI to streamline material replenishment processes and address supply chain challenges effectively. By leveraging AI to detect anomalies and improve data precision, Flowlity is helping organizations improve shortages and stockouts.
Explore the economic impact of poor retail inventory management & a French law on unsold goods, with e-commerce’s ecological shift.
What are the latest e-commerce trends and best practices in e-commerce? Jérémie Serout, Sales Manager at parcelLab is sharing tips and customer stories with us!
Explore how the e-commerce landscape has evolved, driven by increasing complexity, rising consumer expectations, and global competition.